Visualizing the gradient descent method
Por um escritor misterioso
Last updated 26 abril 2025
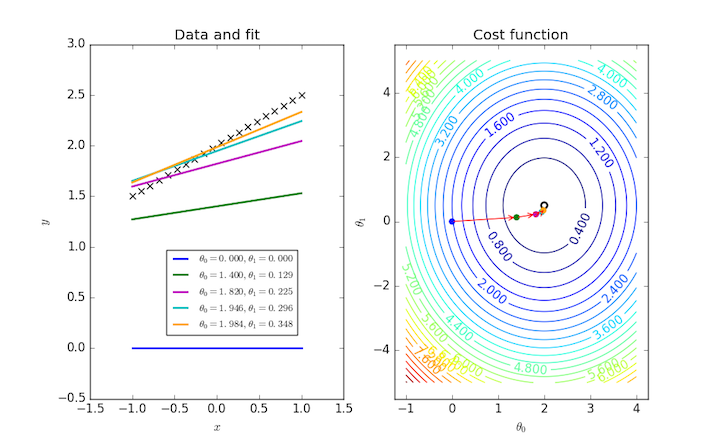
In the gradient descent method of optimization, a hypothesis function, $h_\boldsymbol{\theta}(x)$, is fitted to a data set, $(x^{(i)}, y^{(i)})$ ($i=1,2,\cdots,m$) by minimizing an associated cost function, $J(\boldsymbol{\theta})$ in terms of the parameters $\boldsymbol\theta = \theta_0, \theta_1, \cdots$. The cost function describes how closely the hypothesis fits the data for a given choice of $\boldsymbol \theta$.

How to visualize Gradient Descent using Contour plot in Python
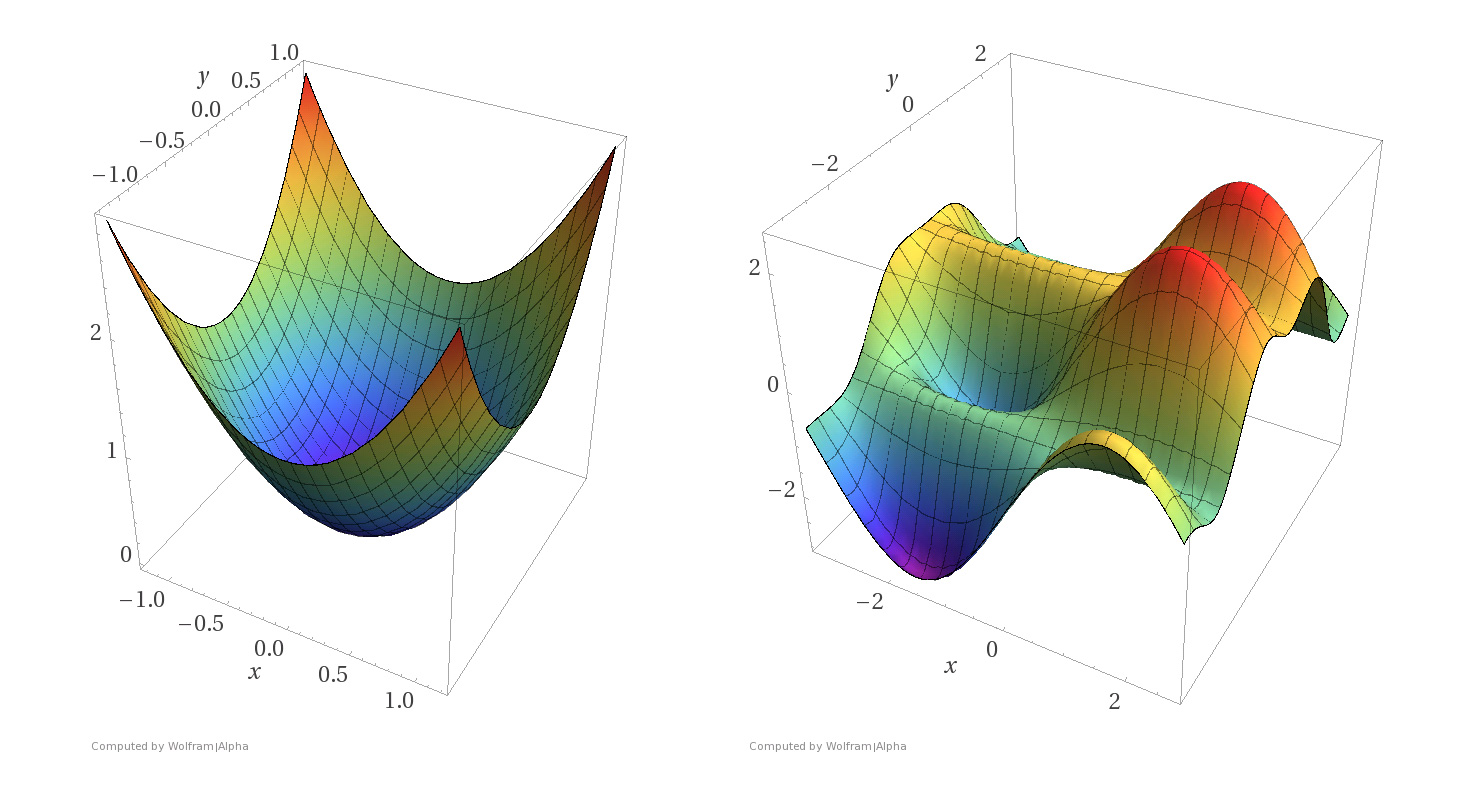
Demystifying Gradient Descent Linear Regression in Python

Simplistic Visualization on How Gradient Descent works
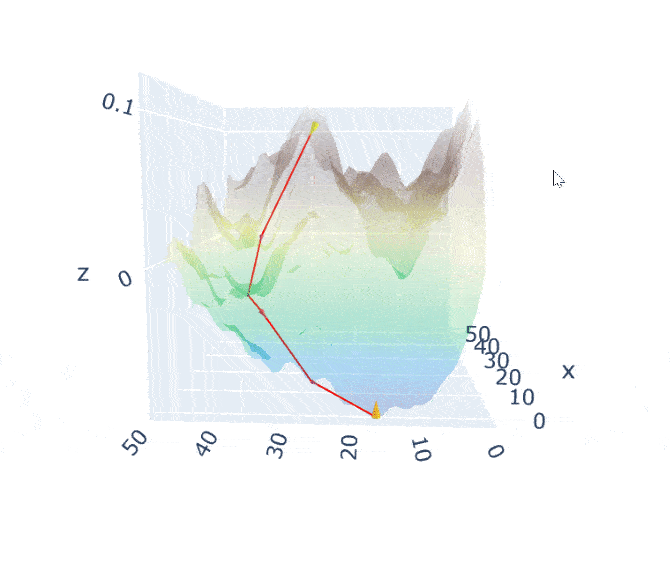
Jack McKew's Blog – 3D Gradient Descent in Python
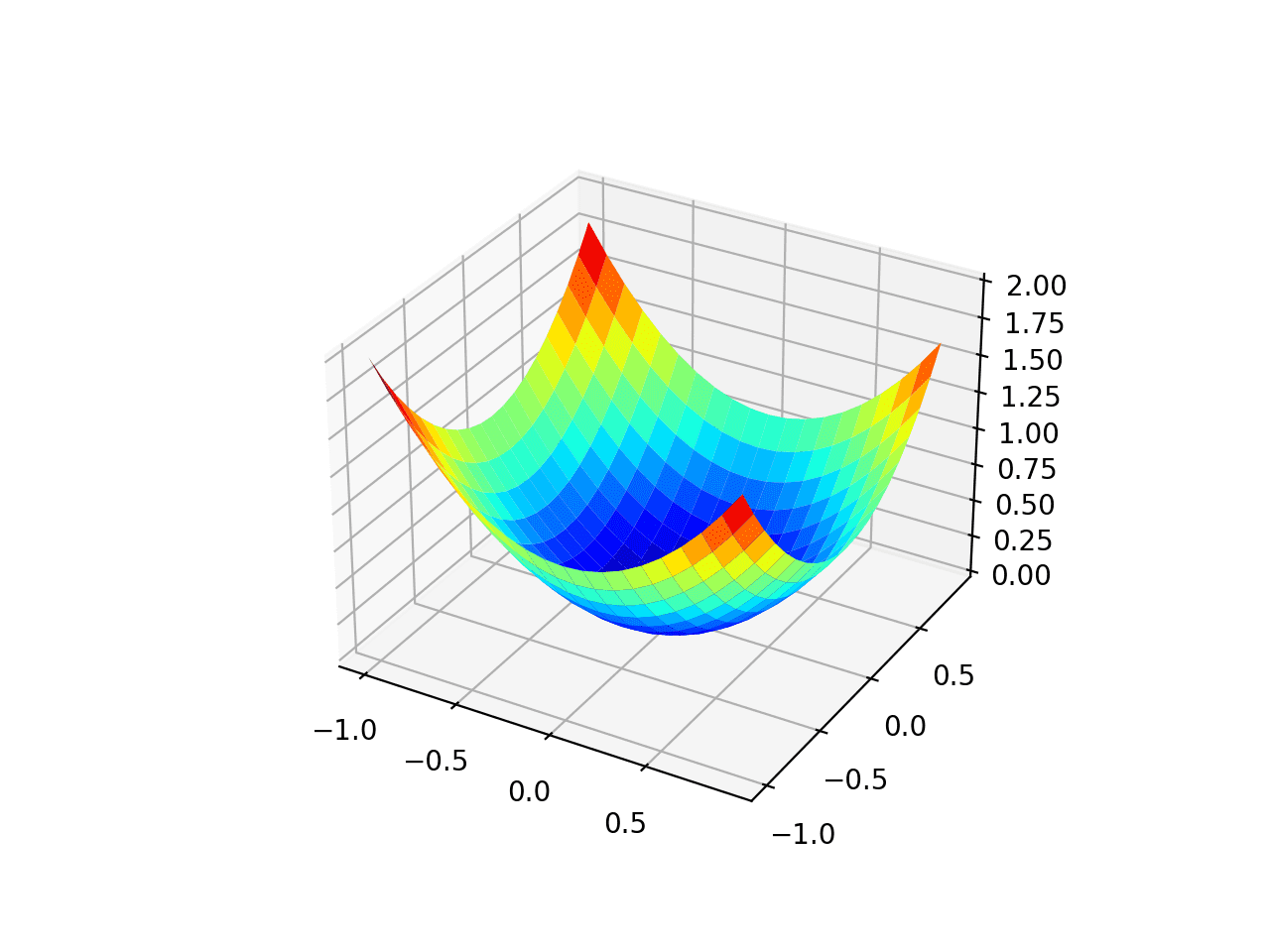
Gradient Descent With AdaGrad From Scratch
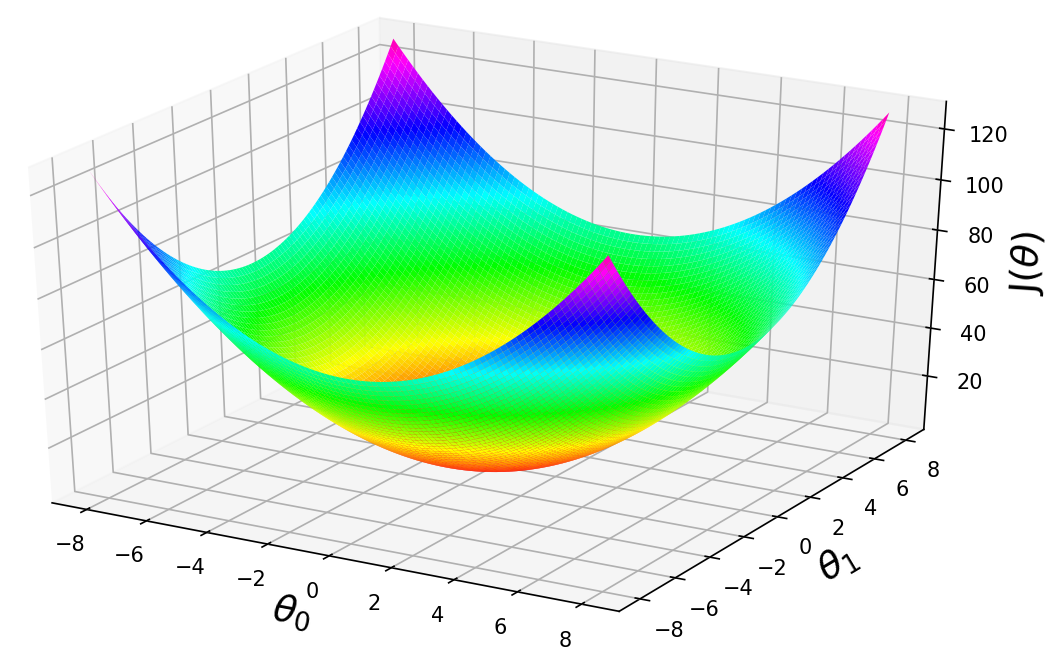
Guide to Gradient Descent Algorithm: A Comprehensive implementation in Python - Machine Learning Space
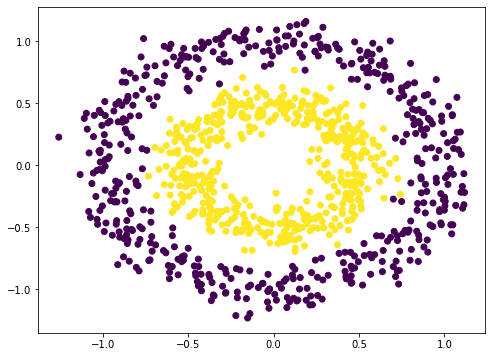
Visualizing the vanishing gradient problem
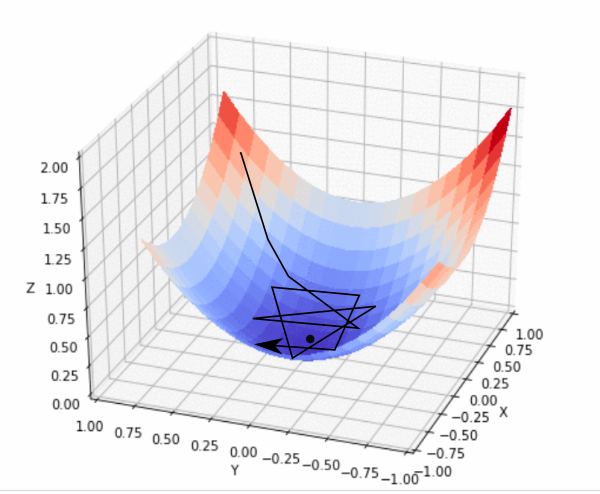
Intro to optimization in deep learning: Gradient Descent
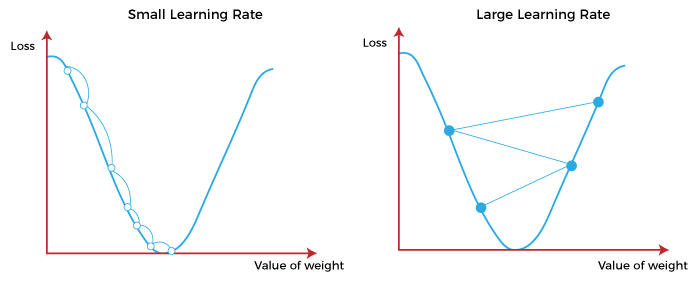
Gradient Descent in Machine Learning - Javatpoint
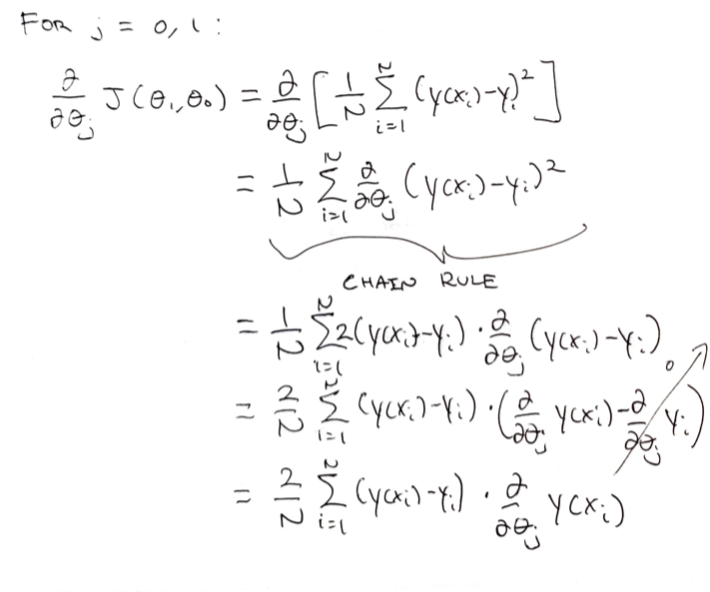
Visualizing the gradient descent in R · Snow of London