Fractal Fract, Free Full-Text
Por um escritor misterioso
Last updated 26 abril 2025
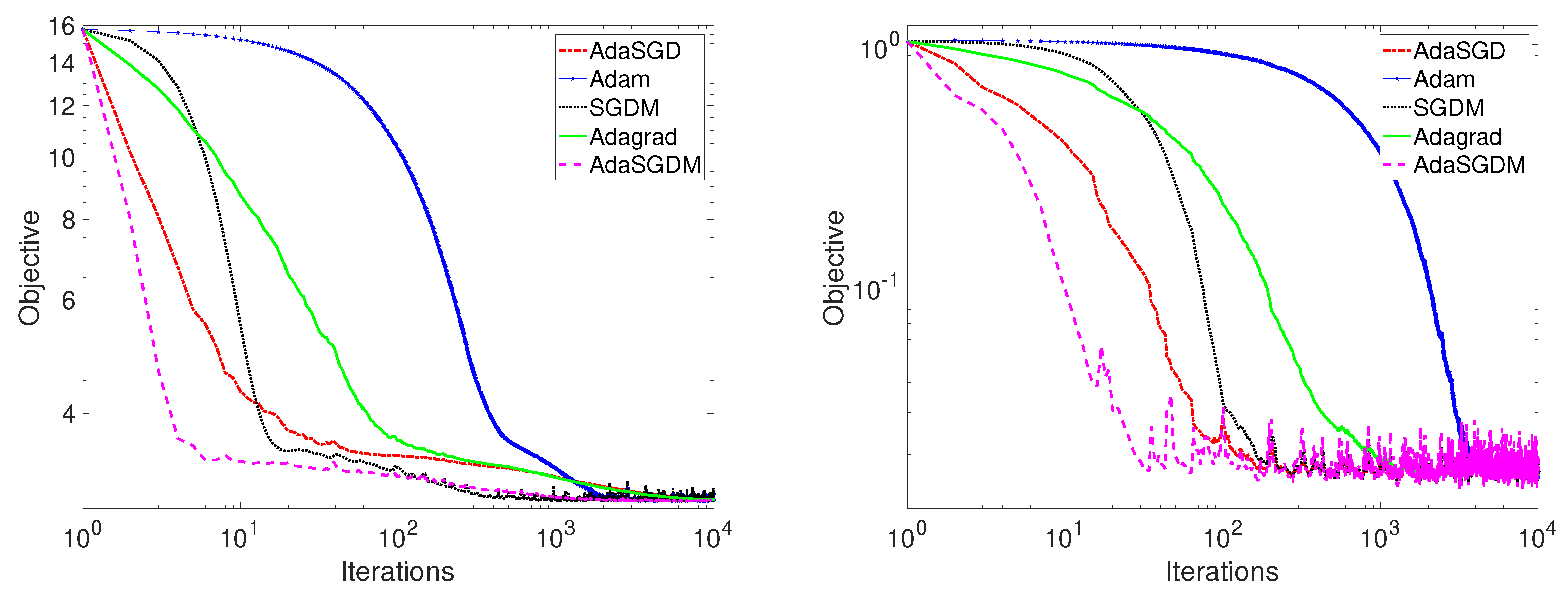
Stochastic gradient descent is the method of choice for solving large-scale optimization problems in machine learning. However, the question of how to effectively select the step-sizes in stochastic gradient descent methods is challenging, and can greatly influence the performance of stochastic gradient descent algorithms. In this paper, we propose a class of faster adaptive gradient descent methods, named AdaSGD, for solving both the convex and non-convex optimization problems. The novelty of this method is that it uses a new adaptive step size that depends on the expectation of the past stochastic gradient and its second moment, which makes it efficient and scalable for big data and high parameter dimensions. We show theoretically that the proposed AdaSGD algorithm has a convergence rate of O(1/T) in both convex and non-convex settings, where T is the maximum number of iterations. In addition, we extend the proposed AdaSGD to the case of momentum and obtain the same convergence rate for AdaSGD with momentum. To illustrate our theoretical results, several numerical experiments for solving problems arising in machine learning are made to verify the promise of the proposed method.
Measure, Topology, and Fractal Geometry

Hi, Today I am posting simple fractal animation in full HD 1920x1080p, this is free background video
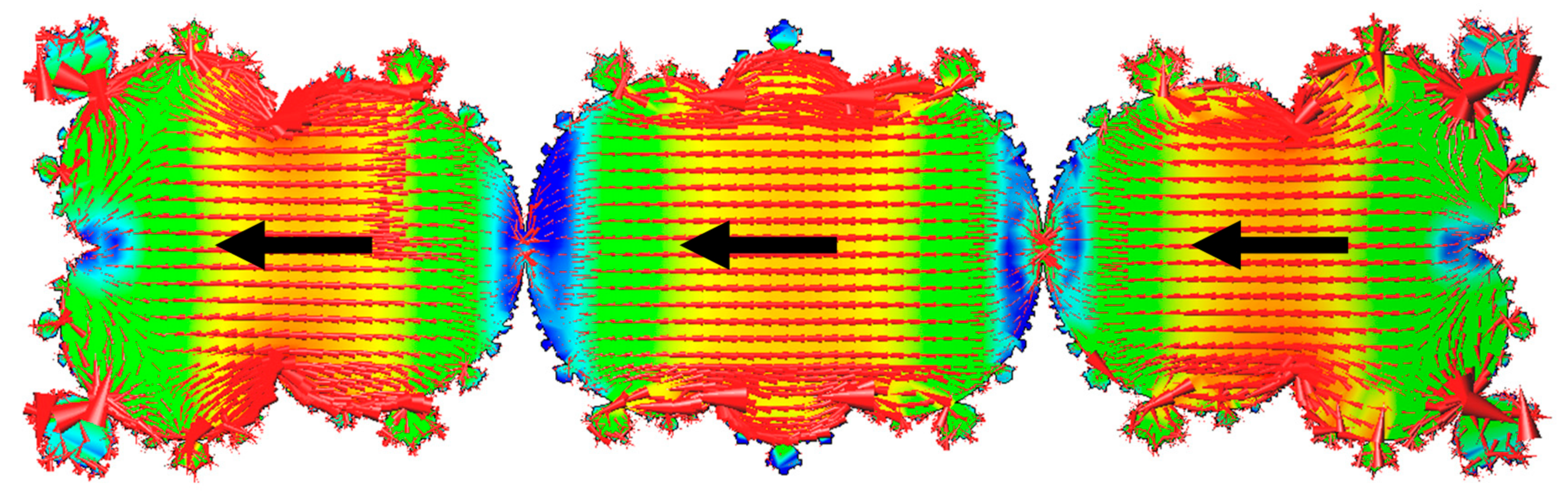
Fractal Fract, Free Full-Text
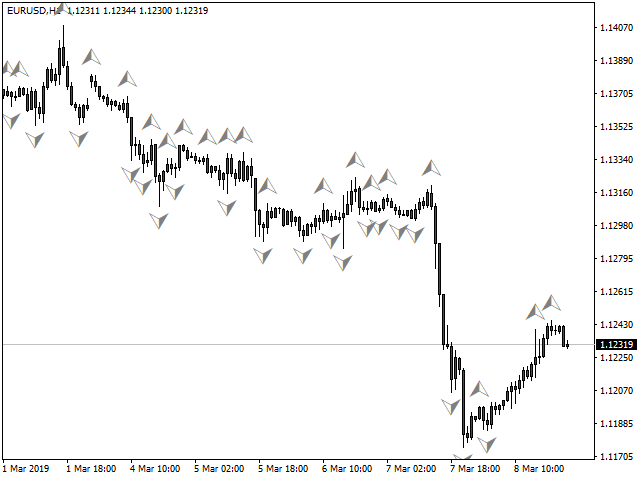
Fractals with Alert for MT4 and MT5

Stock Photo and Image Portfolio by wirow
On the fractal patterns of language structures

How pore structure evolves in shale gas extraction: A new fractal model - ScienceDirect

Fractal Pictures [HQ] Download Free Images on Unsplash
Thermodynamics and Characteristics of Heterogeneous Nucleation on Fractal Surfaces
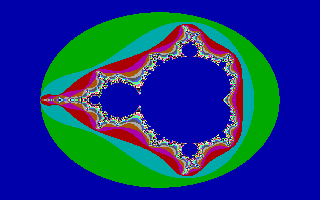
Fractint - Wikipedia